Making decisions is hard. When you choose a restaurant, do you fall back on favorites or try something new? With the regular spots you know what you’re getting, so you’re lowering your chance of regret. But you could be missing out on something better. On the other hand, exploring a new restaurant increases your risk of suffering through sad and soggy scallion pancakes. We call the former behavior “exploitation” and the latter “exploration” -- essentially, exploiting the information you have versus exploring for new data. Exploitation and exploration have to be balanced for you to have a decent shot at sustainable, successful decision-making.
在网络广告中,我们可能会面临为广告活动选择不同的竞价模式或策略的问题。应该如何平衡开发和探索,以达到最佳效果?
We recently published a paper on how to address this problem with our Automatic Model Selector (AMS). It’s a system for scalable online selection of bidding strategies based on live performance metrics. Yes, a human can set up multiple bidding strategies -- but AMS can choose the right one at the right time as needed to maximize performance. It automatically balances explore-exploit.
该系统采用多臂匪徒(MAB),针对实时流量持续运行和评估多个模型,将最多的流量分配给性能最好的模型,同时减少对性能较差模型的流量。它通过向所有模型提供非零流量进行探索,以便对每个模型进行评估,并通过将大部分流量分配给表现最好的模型进行开发。随着时间的推移,开发的程度会增加,因为系统对哪个模型表现最好更有信心。该图给出了AMS系统的组件概览。
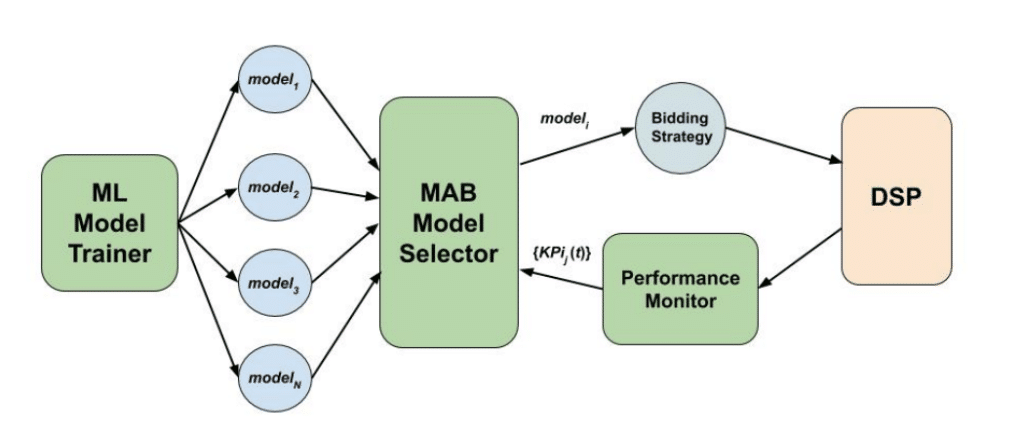
图1.AMS系统的组成部分。ML模型培训师提供训练有素的ML模型作为MAB算法的武器,由MAB模型选择器运行。选定的模型为在DSP上运行的实时RTB活动提供竞价算法。活动的性能关键绩效指标由性能监测器跟踪,在此基础上更新武器的选择概率。模型每15分钟更换一次,绩效关键绩效指标每天更新一次。
与严重依赖人类的传统模型评估过程相比,AMS有一些优势。
- AMS使用您的媒体指标(如CTR、CPC等),定期自动评估在线数据的模型性能。这就避免了依赖旧的或陈旧的数据的可能性,以及媒体指标和机器学习指标之间的不一致。
- AMS是 灵活.它为每个活动单独进行模型选择,而不是选择一个模型并将其应用于所有活动。较简单的模型可能对小型活动有更好的表现,而大型活动可能需要复杂的模型。AMS可以根据具体的广告商或市场情况,找到最适合每个活动的模型。
- AMS是 可扩展. 它通过自给自足地运行受控实验来节省时间,使人们能够专注于高水平的战略。AMS在个案的基础上评估候选模型,并系统地将评估结果应用于转变模型。
这个系统在最初的在线实验中被证明是有效的。 虽然AMS还没有反映在我们的实际产品中,但这项研究正在推动我们对未来创新的思考过程。.如果你有兴趣了解更多关于AMS的细节,或者在线实验的结果,你可以在我们发表的论文中找到相关信息。 使用多臂强盗的在线和可扩展模型选择.